
COVID
LSHTM COVID model
Lead modeller: Yang Liu
Link to all modelling group members
​
Institution(s): London School of Hygiene & Tropical Medicine (LSHTM) and
Brief description of model:
​
The LSHTM-KEMRI team uses CovidM to assess the impact of COVID-19 vaccines. CovidM is a stage-specific dynamic transmission model designed to incorporate complex immune dynamics (e.g. waning of protection to different degrees), to evaluate different vaccine characteristics (e.g. preventing infection, reducing severity), and to generate a range of impact metrics (e.g. cases, deaths, loss of disability-adjusted life years). The model is fitted to country-level reported COVID-19 deaths by mid-2022, where possible, using maximum likelihood estimation and differential evolution optimisation. Differences between countries are driven by (1) demographics (both current and future projections), (2) age-specific contact patterns, (3) local natural history of COVID-19 epidemics (including the influences of public health and social measures), and (4) impact parameters (e.g. life expectancy). The sources of uncertainty around the model results are the model fitting process, epidemic parameters (e.g. rate of immunity waning, severity of future emerging strains), and vaccine efficacy estimates.
​
​
Model code (where available):
​
​
___________________________________________________________________________________________________________________________________________________________________
Imperial COVID model
Lead modeller: Azra Ghani
Link to all modelling group members
​
Institution(s): Imperial College London
Brief description of model:
​
We use an individual based stochastic model as described by Hogan et al [1]. The model consists of two components aimed at understanding both the immunological and transmission dynamics of COVID-19.
Regarding the immunological aspect, our model describes the correlation between neutralizing antibody titres (NAT) and the degree of protection against infection and severe illness (hospitalizations) over time. This model is based on the work from Khoury et al [2] but extends it by deriving immunity levels directly from clinical outcomes such as hospitalization and mortality [3]
To account for hybrid immunity, we adjust NAT levels following each infection similarly to vaccination-induced NAT, assuming they decay at the same rate. Additionally, we introduced considerations for strain-specific protection and breadth of immunity for emerging variants. We achieve this by factoring in a reduction in infection-induced NAT against new variants, mirroring the observed decrease in vaccine-induced protection due to immune evasion.
Regarding transmission dynamics, we use a stochastic, individual-based model of SARS-CoV-2 transmission and vaccination [1]. This model captures key aspects of simpler compartmental models while also introducing hybrid immunity dynamics more efficiently. This comparative alignment with simpler models allows for the integration of relevant fits, while the individual-based model framework enables a more parsimonious representation of hybrid immunity. The model accounts for variations between countries in terms of demography, age mixing patterns, and access to healthcare facilities.
​
​
​
​
​
​
​
​
​
​
​
Figure taken from [1]
​
Fig 1. Schematic representation of the compartmental epidemiology of the stochastic individual-based model. The green circle denotes the IL, which increases in response to both exposure to infection and to vaccination and wanes over time (see section on the Immunological Model). The IL is tracked for each individual in the model and influences the probability of being infected (from susceptible S to exposed E) and of developing disease requiring hospitalisation (Icase) given a breakthrough infection. Imild denotes mild symptomatic disease, Iasymp asymptomatic infection, Ihosp disease requiring hospitalisation (which is comprised of people requiring mechanical ventilation, people requiring oxygen, and people requiring neither), IICU disease requiring ICU admission, Irec stepdown from ICU, and D death. ICU, intensive care unit; IL, immunity level.
​
​
​
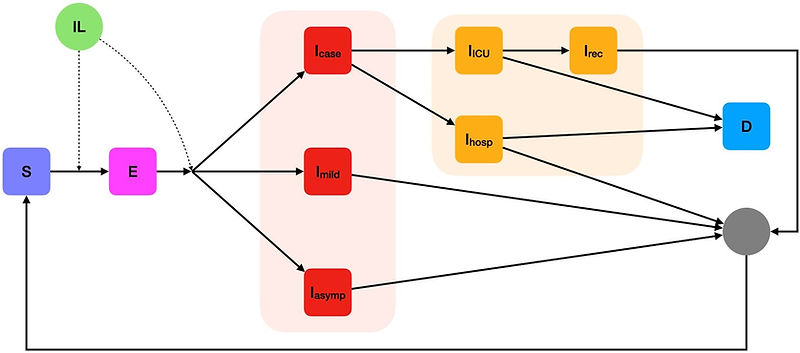
​
Hogan, A.B., et al., Long term vaccination strategies to mitigate the impact of SARS-CoV-2 transmission: a modelling study. PLOS Medicine, 2023.
Khoury, D.S., et al., Neutralizing antibody levels are highly predictive of immune protection from symptomatic SARS-CoV-2 infection. Nature Medicine, 2021. 27(7): p. 1205-1211.
Hogan, A.B., et al., Estimating long-term vaccine effectiveness against SARS-CoV-2 variants: a model-based approach. Nature Communications, 2023. 14(1): p. 4325.
​
​
Model code (where available):
​
​
​